a survey – Bank Underground
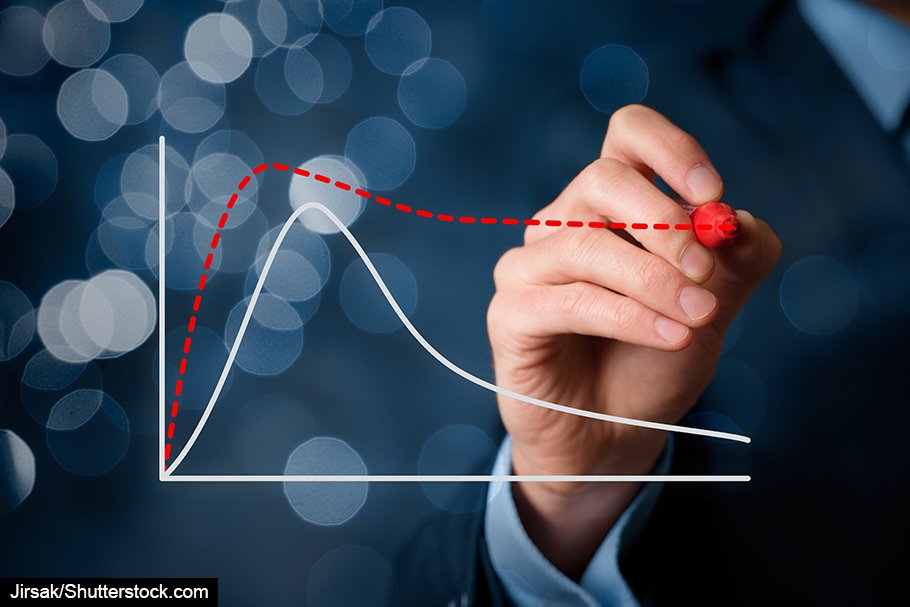
Tihana Škrinjarić

How effective is macroprudential policy and how should policymakers measure its stance? My recent paper surveys the literature on the topic of Growth-at-Risk (GaR), which has been developed as a methodology to provide answers to these questions by relating the effects of macroprudential policy tools to real-economy dynamics. While the results are mixed, the consensus finds a positive impact from macroprudential policy tightening during the expansion of the financial cycle. Policy loosening reduces the potential GDP losses during contractions, with the effects being more prominent in the medium term. Several challenges within this framework still exist. Resolving these would lead to a more accurate evaluation of macroprudential policy effectiveness. Finally, I discuss GaR policy applications.
Background
GaR models link future GDP growth across its entire distribution to current macrofinancial conditions. These models provide a framework for estimating the impact of macroprudential policy actions on future GDP growth across different parts of the growth distribution and across different time horizons, which has direct interpretability for policymakers. This means that the results from GaR models can be useful in informing macroprudential policy, though they do not provide a mechanical guide for setting macroprudential policy.
Initial work such as Adrian et al (2016) and Giglio et al (2015) introduced the GaR concept to policymaking by linking financial conditions to the future dynamics of economic growth, as they have good predictive power in the short term. Subsequently, the literature introduced financial vulnerability indicators to the GaR model, as they have predictive power of possible future medium-term risk materialisation that could spill over to the real economy (Krygier and Vasi (2022); and Plagborg-Møller et al (2020)). GaR methodology has expanded into stress testing as well (Ding et al (2022); and Boucherie et al (2022)), as both are forward-looking frameworks.
Using GaR to inform the macroprudential policy stance
After the global financial crisis (GFC), higher capital requirements were one of the main tools used to increase the resilience of financial systems in many countries. Thus, capital requirements could be observed as an initial attempt to summarise macroprudential policy actions to test their effects. That is why subsequent research extended the original GaR model by including these requirements as an additional variable in the analysis. Several applications are found in Aikman et al (2019a, 2019b), Lloyd et al (2021, 2023), and Boyarchenko et al (2022), who found that that higher capital ratio growth significantly reduces future potential GDP losses but does not have effects on future median growth. This is explained by banks not sacrificing credit provisioning to the economy when building capital as this is built during economic expansions.
As capital requirements are not the only macroprudential policy tool, there have been several approaches to aggregating and collating information on macroprudential policy tools in general. Research today utilises several comprehensive databases of macroprudential policy tools, where the full macroprudential policy indicator is the most commonly used indicator. This full version captures all tools that the policymaker has applied, regardless of the scope or the magnitude of the measure, as long as they had a macroprudential nature and have the goal to mitigate systemic risk. The indicator is constructed by counting the number of tools that have been (de)activated, or fine-tuned, and a distinction is made if the tool is of tightening or loosening nature (see Budnik and Kleibl (2018)). The policy indicator can be decomposed based on capital versus borrower-based measures.
Several studies find significant results of macroprudential policy effectiveness by using this type of indicator. Brandão-Marques et al (2020) found overall evidence of policy trade-offs regarding lowering mean future growth (costs) and decreasing the potential future worst losses (benefits): macroprudential policy lessens the impact of presently looser financial conditions on future downside risk of real growth, however, it does come at some cost of losing median growth in the short and medium term. Galán and Rodríguez-Moreno (2020) and Galán (2020) find similar results, and on top of that, the authors found different effects in different phases of the financial cycle. There exists a positive impact from macroprudential policy tightening during expansion of the financial cycle, ie increasing the resilience of the financial system reduces the downside risks to future GDP growth, whereas macroprudential policy loosening has a positive effect on GaR during the contractions. Overall, the effects were more prominent in the short term. Fernández-Gallardo et al (2023) found that macroprudential policy affects the composition of credit: tighter macroprudential policy is effective at preventing both household and business credit booms. Furthermore, borrower-based measures are found to be the ones that seem to have more common significant effects so far.
Analysis of policy interactions has been on a rise as well. Perhaps the most interesting analysis is on the interaction between monetary and macroprudential policy, as both have implications for future growth. As an example, the empirical results of Duprey and Ueberfeld (2020) show that macroprudential tightening is more effective in reducing downside risks of future growth compared to monetary policy tightening.
Despite these findings so far, I identified some challenges that still need to be resolved: how to define and measure the macroprudential policy variable; endogeneity of the variable, as economic policies react to observations they make about the economy and financial system; term structure of the estimated effects, ie when certain tools have significant effect; and some data unavailability issues. Below I comment on one that is important from the policymaker application perspective, others are examined in the survey paper.
Measurement of the policy variable is a challenge by itself, due to many policy instruments, of which some are broad-based, and others are targeted at specific vulnerabilities. Some instruments are actually of a non-macroprudential nature that were adapted into macroprudential purposes after the GFC. There is also a difference between the effects of a tool being introduced for the first time, versus later fine-tuning of the instrument itself. One example is to give greater weight or value to a tool activation, compared to changing the level of the tool. Moreover, the scope of a measure also has different effects, as some macroprudential measures can affect both the borrower and lending side, whereas others effect just the lending side.
Policy applications
Initial findings in the literature indicate that there can be a trade-off when using macroprudential policy between reducing future GDP-at-Risk and lowering the mean outcome for GDP growth (Galán (2020)). However, other authors do not find negative effects on mean growth at all (Fernández-Gallardo et al (2023)). Moreover, net benefits (in term of comparing costs versus benefits) are realised in the medium term (Brandão-Marques et al (2020); and Boyarchenko et al (2022)). These effects also vary with respect to the position within the financial cycle, with borrower-based measures being a bit more effective. Thus, the framework itself can be used to evaluate the optimal timing of macroprudential policy instruments.
In order to do so, the GaR framework approach could be complemented with more granular data analyses, as it cannot provide information on the transmission mechanisms of the policy itself. As granular data becomes more available, macroeconomic approaches such as GaR can be supplemented with a micro approach, ie analysing how changes of macroprudential tools are reflected in bank behaviour. In that way, policymakers could base the calibration of instruments on information about the timing and ways some tools have effects. The choice of the timing could reflect the transmission lags of macroprudential policy instruments into specific variables, such as bank lending (see Davis et al (2022)), and other relevant variables of interest.
Tihana Škrinjarić works in the Bank’s Stress Testing and Resilience Division.
If you want to get in touch, please email us at bankunderground@bankofengland.co.uk or leave a comment below.
Comments will only appear once approved by a moderator, and are only published where a full name is supplied. Bank Underground is a blog for Bank of England staff to share views that challenge – or support – prevailing policy orthodoxies. The views expressed here are those of the authors, and are not necessarily those of the Bank of England, or its policy committees.
Share the post “Growth-at-Risk for macroprudential policy stance assessment: a survey”